Introduction
Artificial Intelligence (AI) systems are becoming more integrated. This interconnection generates new opportunities while simultaneously posing obstacles and threats. For example, if a doctor utilizes AI to expedite the medical clearance procedure, but the insurance company’s AI rejects it for unknown reasons, delays and dissatisfaction may ensue. In these instances, two AI systems are unable to “talk” to each other efficiently.
To effectively manage such AI systems, firms must train personnel, strengthen technical cooperation, and establish robust governance frameworks that assure responsibility, trust, and safety. Here’s how firms can tackle these difficulties.
Training individuals to work with AI systems.
AI systems are widely employed throughout sectors, thus it is critical for employees to understand how to deal with them. But merely learning how to utilize AI technologies isn’t sufficient. Employees must also grasp how various AI systems communicate with one another.
For example:
In healthcare, an artificial intelligence system that forecasts organ transplant success rates devalued younger patients since its projections were limited to five years.
In banking, credit scores generated by one AI system may have flaws that influence downstream systems, increasing bias.
In law, AI technologies aid in legal research, but attorneys must guarantee that these tools integrate smoothly and that their findings are explained to clients.
Proper training should assist employees:
Evaluate AI results critically.
Understand how the data from one system affects the data in another.
Identify and resolve issues that arise when AI systems interact.
To avoid misdiagnosis, physicians should match AI results to patient data. Similarly, specialists should be able to identify and eliminate biases that run via AI systems.
Improving AI Coordination with Technology.
AI systems can “talk” to one other and collaborate more effectively with the assistance of technology. The idea is to create AI frameworks that coordinate systems at every level, from data collecting to final conclusions.
For example:
In healthcare, an AI system might process radiological data (upstream), tailor it to hospitals (midstream), and then aid clinicians (downstream). If these layers are not aligned, mistakes will propagate throughout.
Artificial intelligence algorithms that detect hazardous material and track user activity on social media sites may struggle to communicate effectively. This can result in gaps, such as neglecting to report unlawful content.
To address this, enterprises must develop linked AI frameworks from the outset. For example, healthcare systems may guarantee that diagnostic AI technologies communicate easily with triage systems.
Companies can also utilize “adversarial AI,” or AI that tests other systems, to identify dangers early. Businesses may increase overall productivity, decrease risks, and avoid errors by synchronizing AI systems.
Creating AI Governance Frameworks
The complexities of AI ecosystems necessitate good governance to avoid cascading mistakes, biases, and accountability gaps.
Effective governance focuses on:
Transparency: If an AI tool makes a mistake, businesses must track the issue back to its origin. Was there a data issue, a model failure, or an implementation issue?
User Empowerment: Employees and end users should be able to tailor AI systems to their specific demands while adhering to safety protocols. For example, clinicians can tailor AI technologies to their particular patient group, increasing accuracy.
Feedback Loops: AI outputs should be regularly monitored for conflicts and mistakes.
Regulations such as the EU’s Digital Services Act (DSA) provide criteria for assuring AI transparency, accountability, and user empowerment. Businesses may use similar ideas to establish trust with their workers, customers, and regulators.
For example, hospitals may use traceability frameworks to justify diagnostic decisions, while internet platforms can employ audit tools to moderate content.
Conclusion: Understanding the Future of AI Ecosystems
As organizations depend more on AI, maintaining networked systems will grow more difficult. Success depends on:
Employees will be trained to operate with numerous AI systems.
Using technology to align and coordinate artificial intelligence technologies.
Developing governance structures that promote accountability, adaptation, and transparency.
By using these principles, firms may utilize AI to improve workflows in a safe and effective manner while reducing risks.
Authors: I. Glenn Cohen, Theodoros Evgeniou, and Martin Husovec. Published on December 16, 2024. You can check out the full article here.
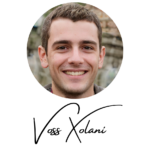
I’m Voss Xolani, and I’m deeply passionate about exploring AI software and tools. From cutting-edge machine learning platforms to powerful automation systems, I’m always on the lookout for the latest innovations that push the boundaries of what AI can do. I love experimenting with new AI tools, discovering how they can improve efficiency and open up new possibilities. With a keen eye for software that’s shaping the future, I’m excited to share with you the tools that are transforming industries and everyday life.