DeepMind, Google’s AI research unit, has developed a breakthrough way for training artificial intelligence (AI) models. This new approach, known as JEST (Joint Example Selection), dramatically increases the speed and energy efficiency of AI training. According to DeepMind, JEST can boost performance by 13 times and power efficiency by ten times over existing approaches.
How JEST works
Traditional AI training methods rely on individual data points, but JEST trains on whole batches of data. Here’s a brief explanation of how JEST works: First, a smaller AI model is developed to evaluate the quality of data from high-quality sources, with data batches ranked depending on quality. The graded high-quality data is then contrasted with a larger, lower-quality dataset. The smaller model chooses the optimal data batches for training. Finally, the smaller model’s results are used to train a larger model. JEST optimizes data selection so that only the best data is utilized for training, resulting in faster and more efficient learning.
The advantages of JEST
DeepMind’s study identifies three important advantages of the JEST technique. First, JEST takes up to 13 times fewer training iterations and utilizes 10 times less processing resources than other cutting-edge models, making it very efficient. Furthermore, training procedures are greatly expedited, resulting in noticeable speed increases. Finally, with its greater power efficiency, JEST can lessen the environmental effect of AI data centers, resulting in significant energy savings.
Challenges and Limitations.
However, the performance of JEST is strongly dependent on the quality of its training data. To work well, the approach requires a well-curated, high-quality dataset. The “garbage in, garbage out” concept states that without high-quality beginning data, the benefits of JEST cannot be achieved. As a result, amateur AI engineers may struggle to execute the approach, which requires expert-level data curation abilities.
Environmental Impact
The adoption of JEST comes at a vital time, as worries about the energy usage of AI systems grow. In 2023, AI workloads required around 4.3 gigawatts of power, which is almost similar to Cyprus’ yearly power usage. With AI’s power needs projected to grow, solutions such as JEST might play an important role in reducing their impact.
For example, a single request to AI models like ChatGPT consumes 10 times more energy than a Google search. According to projections, by 2030, AI might use a quarter of the US electricity system. The JEST technique has the potential to reduce these power needs by improving the energy efficiency of AI training.
Future Implications
The adoption of JEST by large AI players is still unknown. Training big models, such as GPT-4, is expensive, with costs ranging up to $100 million. Future versions could cost even more. Companies may use JEST to maintain or improve training productivity while lowering power usage and expenditures. However, there is a chance that corporations may continue to seek for maximal training output, employing JEST to get quicker outcomes rather than lowering energy use.
In essence, Google’s DeepMind has created a ground-breaking AI training approach that promises huge speed and efficiency gains. The JEST technique might assist solve AI systems’ increasing power consumption, thereby helping both the business and the environment. However, its effectiveness is dependent on the availability of high-quality training data and acceptance by large technology companies.
Original Source: Dallin Grimm, “Google claims new AI training tech is 13 times faster and 10 times more power efficient — DeepMind’s new JEST optimizes training data for impressive gains,” You can check out the full article here.
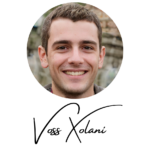
I’m Voss Xolani, and I’m deeply passionate about exploring AI software and tools. From cutting-edge machine learning platforms to powerful automation systems, I’m always on the lookout for the latest innovations that push the boundaries of what AI can do. I love experimenting with new AI tools, discovering how they can improve efficiency and open up new possibilities. With a keen eye for software that’s shaping the future, I’m excited to share with you the tools that are transforming industries and everyday life.